Why Political Parties are Bothered about Social Media Sentiments? Has Technology Played a Role Complex Machine Learning (ML) algorithms can be used to automatically assign a mood label to individual posts depending on the purpose of analysis
Opinions expressed by Entrepreneur contributors are their own.
You're reading Entrepreneur India, an international franchise of Entrepreneur Media.
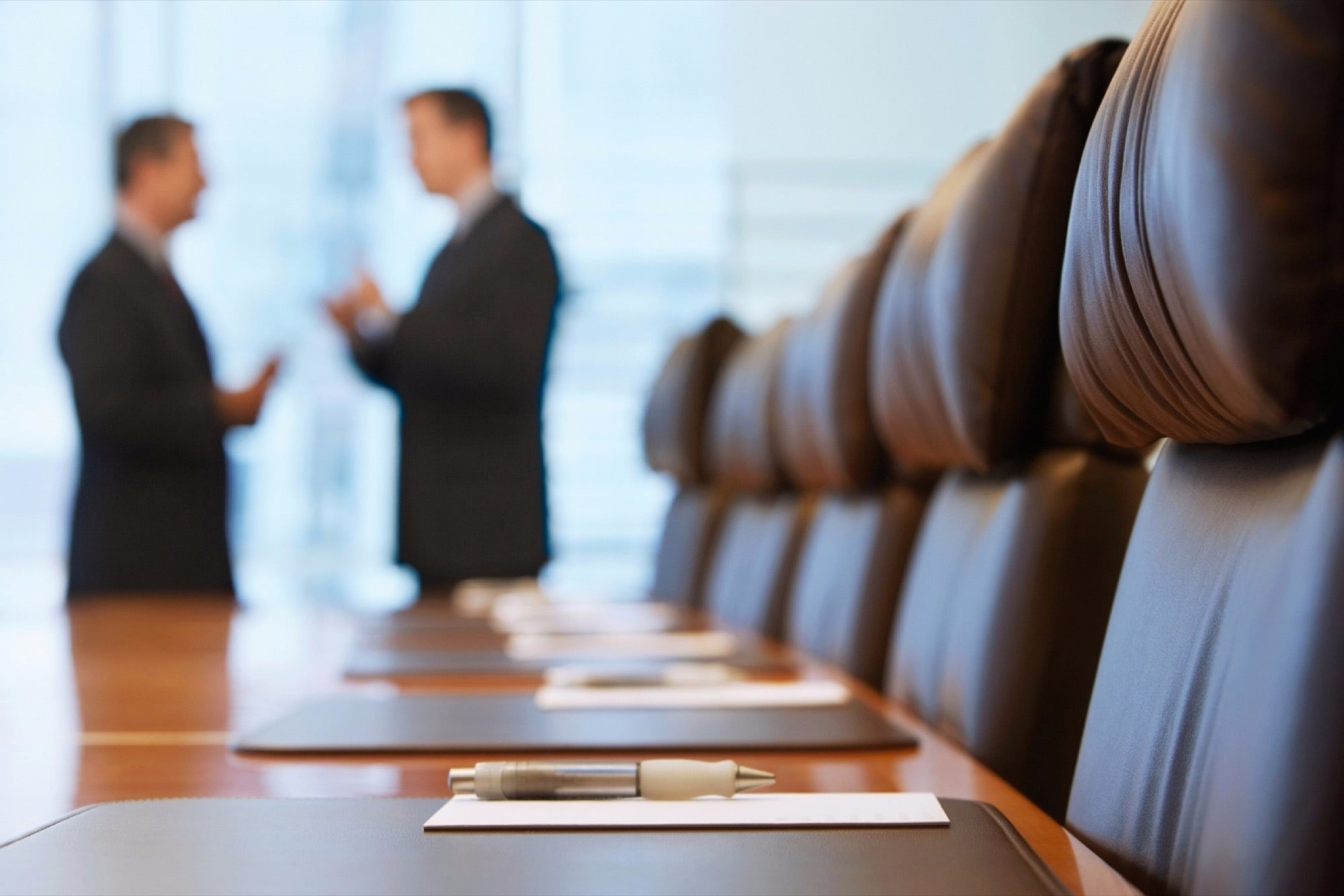
The ongoing elections in India have conclusively demonstrated how seriously all political parties take social media as a modern communication tool. The more progressive ones have also grown comfortable with using social media for gauging the public mood on a real-time basis and undertaken course corrections in the form of public statements or policy changes basis this judgment.
What is popularly known as social media opinion monitoring or sentiment analysis typically involves an algorithmic or manual analysis of real-time social content in the form of tweets and Facebook posts. Complex Machine Learning (ML) algorithms can be used to automatically assign a mood label to individual posts depending on the purpose of analysis. For example, a typical mood label can identify a tweet as positive, negative or neutral, and another label can further drill down a positive tweet as hopeful, joyful, grateful etc. Smarter analysis often involves constantly revisiting and reviewing the mood labels in the context of an emerging situation.
The Way it is Done
Once posts are categorized, the macro-level analysis helps political parties assess the overall mood of users on any trending political issue, whereas the drill-down analysis can identify deeper sentiments or specific concerns. A thorough analysis can even feed into the communication engines run by parties as they can target all individual users identified with a particular mood label with specially designed messages on social or digital channels.
Consider a recent example where one political party in India accused another competitive party of using military vessels for personal holidays, which sparked a major social media controversy with several hundreds of thousands of people expressing their opinions online in a short period of time. On the second day, the accused party countered the other party of paying a dirt-cheap fare to misuse military aircraft for ferrying its leaders for political rallies. This ensured that the Indian social media remained hyperactive for the second consecutive day with various expressive tweets and updates.
Meaningful Insight
A sentiment analysis done by either party could have revealed that accusations of one party were taken more seriously than the others by the social media users. Whether found favourable or unfavourable, either party could then alter their campaign messaging to better align with the public mood on a real-time basis.
However, in order to generate meaningful and actionable insights, it is imperative that political parties or companies use caution while planning and conducting sentiment analysis. Perhaps the most important consideration is about choosing the correct analysis algorithms, which can either be human-led or machine-powered. While the advancements in Natural Language Processing (NLP) have made it possible to get highly accurate results, the human-led sentimental analysis will continue to be the last word in accuracy for the foreseeable future. The high volume of data or a short deadline is the only two limiting factors for human-led analysis; whereas sarcasm detection remains a weak point for machine-powered analysis.
Secondly, choosing the correct dictionary repository is very important for getting accurate results. There are words which can have different emotions in two different contexts; for example, the word flamboyant denotes a positive emotion in the fashion industry, however, it would carry a negative emotion when used in relation to a government minister or an officer. There are different dictionaries for different contexts and an appropriate one should be chosen or custom created if necessary.
Thirdly, it is more important to consider negative opinions first. One negative statement can start a chain of events leading to a negative spiral which can have lasting damage on a politician's image. It is therefore critical for parties to take immediate corrective action as soon as they sense that the public sentiment of masses is turning negative.
Last but not least, consistency is the key. Sentiment analysis must be an ongoing and continuous activity that will not only train the ML algorithms and increase their accuracy but also allow decision-makers to respond to fast-shifting public sentiment effectively and proactively. Done effectively, sentiment analysis can be a highly useful alternative to ears-on-the-ground tactics that politicians and rulers have used since the dawn of time.