The Importance Of Having Accurate Facial Recognition Faulty face recognition technology can potentially lead to lawsuits and damage brand reputation
By Kishore Natarajan •
Opinions expressed by Entrepreneur contributors are their own.
You're reading Entrepreneur India, an international franchise of Entrepreneur Media.
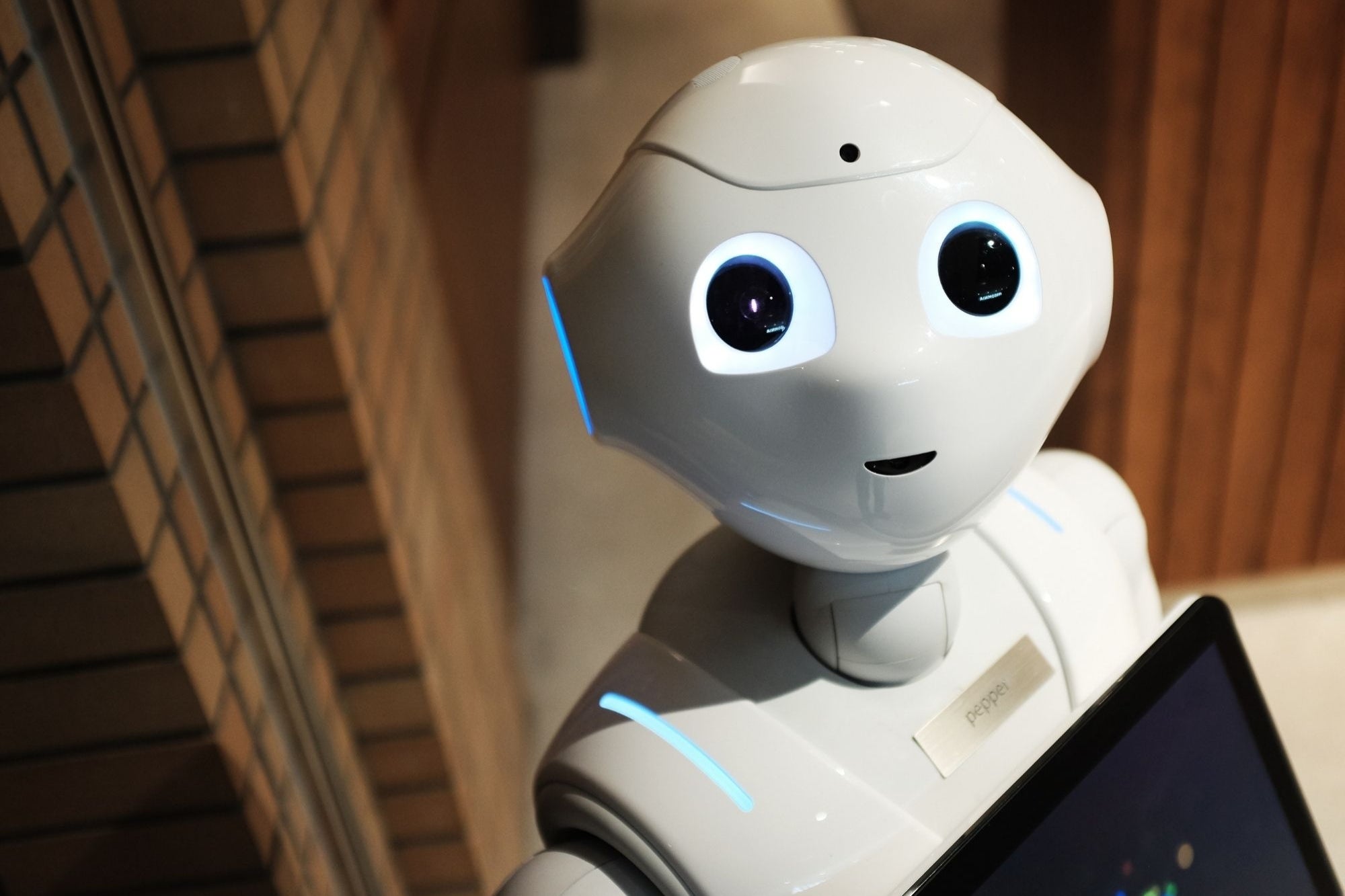
Not too long ago, unions in the UK claimed Microsoft's face recognition software Face API failed to recognize black drivers accurately. This caused mayhem for several Uber drivers as the misidentification resulted in illegal deactivation of their accounts. This is called racial bias in AI. This made the government and authorities ponder if facial recognition was reliable and whether it must be used at all.
Today more than ever facial recognition has gained significant importance. Owing to the global COVID-19 outbreak, the use of facial recognition received high traction as it was a boon to provide uninterrupted and contactless services to consumers for various use cases.
The adoption of facial recognition is widespread and can be experienced in various parts of our lives such as unlocking phones, customer onboarding/KYC, issuing of identity documents, border and police checks, access control at workplaces, touchless airport security clearance, preventing retail crimes, etc. Broadly, facial recognition systems are being used for either verification or identification and hence it is necessary to make sure that facial recognition systems are extremely accurate.
The accuracy with which an AI system can deliver results defines the system's superiority and sets it apart. Facial recognition (FR) AI has improved dramatically over the last few years. Increasing accuracy of FR systems will help in reducing risks related to misidentification. Many AI systems still use open source, outdated libraries but to ensure accurate results it is necessary that systems use AI libraries that are constantly updated. Depending on how robust and well-maintained the libraries are, the accuracy of the system will be impacted.
For face recognition AI systems to work effectively, factors like consistency in lighting and positioning, Internet connectivity, and image processing are required to work in tandem. The way facial recognition engines are built and the data they are trained with, the quality of images used play an important role in determining the accuracy of the results obtained.
Facial recognition is being used extensively by companies in the Fintech, Crypto, and Gaming sectors to onboard customers. Customer onboarding is an important step that cannot be skipped. Every person who wants to use the company's service must go through it. This includes several aspects of face recognition such as liveness detection and face match. It is necessary that these organizations have a hassle-free and seamless process to onboard consumers as this experience can even affect user conversion and retention depending on how user-friendly the software is. Accurate AI/ML models help differentiate between genuine consumers and fraudsters very quickly.
For businesses that handle few identity verification requests a minute, low or high accuracy AI engines don't make much of a difference. But as the businesses scale and handle hundreds of requests a minute, accuracy becomes a crucial factor. For businesses who look at hundreds of consumer profiles a day, low-accuracy AI engines lead to a decrease in straight-through processing rate and need manual intervention to make decisions, leading to an increase in their corresponding operational cost.
Low face recognition AI accuracies also leads to rising costs for businesses using them. Customers today require and expect quick and efficient services, right from the onboarding process to the final purchase. It is necessary that the onboarding of the customer is smooth, quick and hassle free to convert and retain the customer. It has been observed that those businesses who have complicated or inaccurate facial recognition technology lose consumers which directly impacts the business revenue.
Faulty face recognition technology can potentially lead to lawsuits and damage brand reputation. Additionally, if the primary use of the FR AI technology is for fraud detection and if it fails to deliver accurate results this would lead to multiple frauds taking place and further contributing to revenue losses.
In recent years, a lot of work has been done by scientists and researchers to increase the reliability of face recognition systems, especially to remove racial bias. The level of racial bias depends on how the AI engines are built and trained, not just how good the images being used are. In the future, we should be able to see highly accurate face recognition models that don't have any kind of bias.
It is vital for businesses to make sure that they consider AI accuracy while choosing a face recognition solution to provide their customers with a seamless experience and increase their business revenue. Without extremely accurate AI/ML, it is almost impossible to get consistent results in face recognition.