Pandemic Lessons: What Science Learned From COVID-19 And How Big Data Can Change Medicine Pandemic Lessons: What Science Learned From COVID-19 And How Big Data Can Change Medicine
Opinions expressed by Entrepreneur contributors are their own.
You're reading Entrepreneur India, an international franchise of Entrepreneur Media.
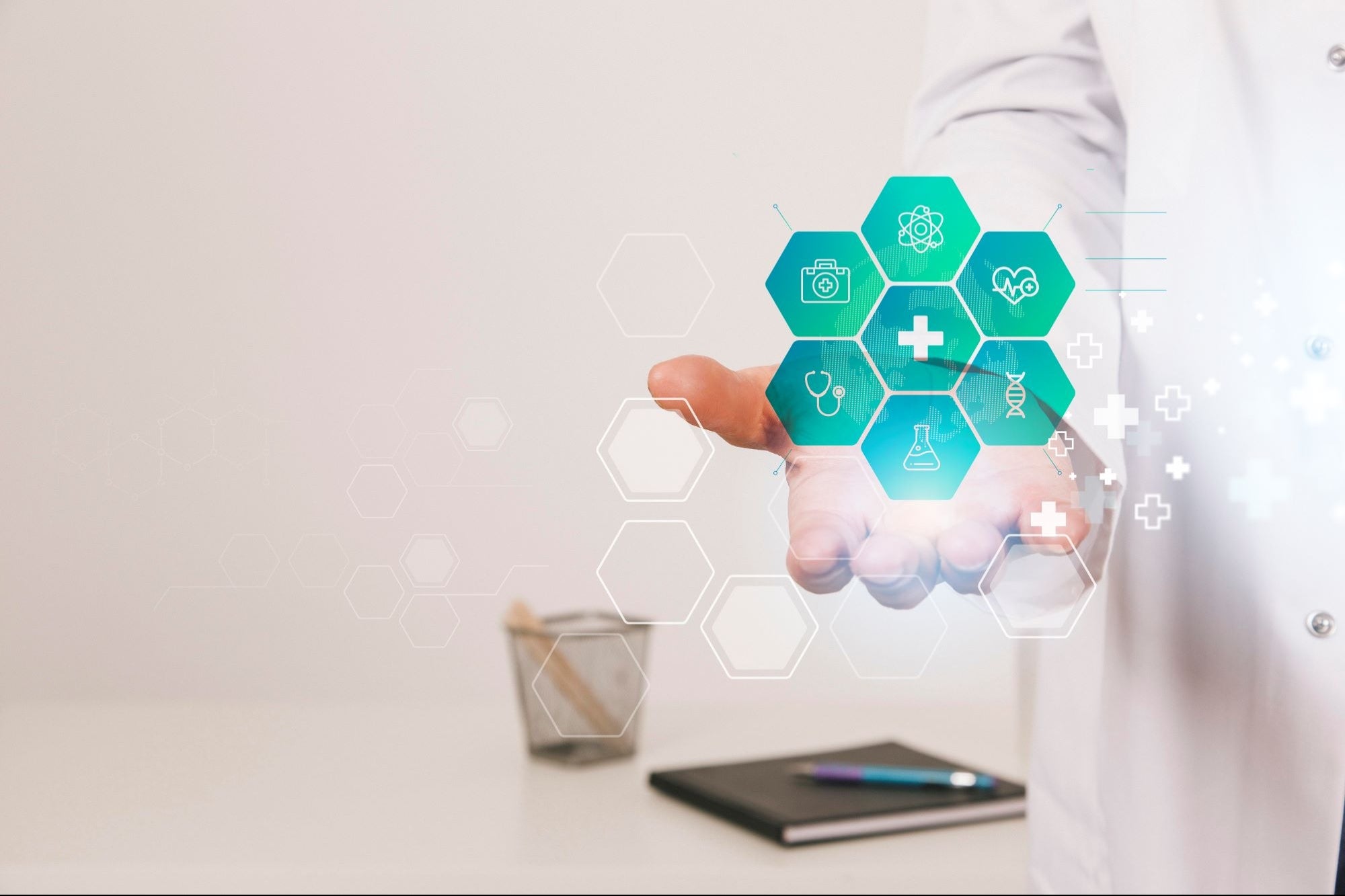
The coronavirus pandemic that broke out in late 2019 and claimed over 6 million lives uncovered many problems in global healthcare, such as widespread unpreparedness of the infrastructure for mass infections, lack of hospitals and medical staff, etc. Instead of prevention and anticipation, officials, epidemiologists, infectious disease specialists and virologists were forced to act in the middle of an ongoing unfamiliar crisis.
Many lessons were learned over the past two years. For example, we realized that any organizational decision (be it lockdowns, COVID hospital deployments, or vaccine development) requires digitized global data on infection spread.
Pandemic analytics
COVID-19 triggered a rapid increase of available digital data that governments can rely on when taking epidemiological measures.
However, even before the epidemiologists were well aware of how infectious diseases can spread worldwide via intercontinental air traffic. SARS, H1N1, MERS, Ebola, Zika virus, and then COVID-19 were quick to find globalization's Achilles' heel: fast travel and frequent social contacts.
Given the high velocity of virus spread and lethality of its first strains, soon it became clear that nations needed to stand together against the new challenge and ensure active and transparent data exchange. At first, that information mainly covered the movements of infected patients; later, countries were sharing the data on infection and death rates, tests, and vaccinations.
The speed and size of available data seem to make COVID-19 stand out among other infections of the 21st century. The information generated day by day quickly became big data, assessed by scientists by the five Vs quality criteria: volume, velocity, variety, veracity, and value.
Researchers emphasize that big data itself has nothing to offer without technologies and practical application. It is the structured information accumulated on a specialized platform or other AI tool that matters and can assist officials, scientists, and medics with their decision-making. For example, it can help epidemiologists analyze waves of infection and make forecasts for the upcoming month. In other words, it provides opportunities for pandemic analytics.
Intelligent analysis of such an unprecedented amount of data, in this turn, would be impossible without high-performance computing resources (such as cloud computing), advanced ML algorithms, and neural networks.
Traffic control in China
Countries that took the first hit of the coronavirus were forced to pioneer the development of analytical tools for monitoring the situations.
As doctors had no understanding of how to treat the new disease, keeping the infection under control by searching for "patients zero" and eliminating transmission-hot zones became the highest priorities. In China, where COVID-19 originated, the initial focus was put on tracking people's mobility via big data and AI technologies.
GeTui, an IT developer, created a heat map of transmission density to track people traveling from Wuhan, the hardest-hit city. On Chinese social media, it was even possible to get information on buses infected persons had taken and people they could have contacted before their hospital admission. Later, a big data analysis platform was launched to consolidate the data provided by the National Health Commission, the Ministry of Transport, China Railway, and the Civil Aviation Administration of China and help the authorities find close contacts of COVID patients and people suspected of having the virus.
Baidu, another major IT company, developed a map that highlighted roadblocks and crowded places. Thanks to real-time updates, its users could plan their routes and reduce the number of potential contacts.
Supervising EU lockdowns
When the coronavirus eventually spread all across the world, epidemiologists placed their bet on minimizing social contacts and imposing restrictions, such as isolation, social distancing, face masks, and remote work. Compliance with lockdown rules in European countries could hardly be controlled without AI technologies.
The Italian Ministry of Innovation, in collaboration with the University of Pavia, adopted big data analytics to their needs, with the government starting to collect anonymous information on user movements from Facebook and Italian IT companies. Later, Enel X energy company and Here Technologies, a mapping content developer, created the City Analytics map that enabled Italian transportation agencies to track passenger mobility.
The French government went even further in their masking campaign and armed themselves with DatakaLab, the AI-based video analytics tool that informed local transportation authorities on maskless passengers. AI Hub, a Singapore tech company developed the SafeDistancer app to monitor social distancing, based on AI and computer vision technologies. The app processes smartphone images to detect people in the camera frame and emits user alerts when people come too close together.
Infection waves and empty hospital beds
It would be strange if COVID-era AI solutions were used only for upholding restriction adherence and monitoring. As the pandemic progressed, countries began to adopt tools that cloud optimize the workload on medical facilities overwhelmed with admissions.
The UK's National Healthcare Service (NHS) partnered with Microsoft, Amazon Web Services, Google, Faculty, and Palantir to introduce a digital platform based on big data, AI, and cloud computing. The platform consolidated the data on COVID patients' length of stay and hospital occupancy level, collected from Public Health England and the NHS. The platform also provided recommendations on hospital staffing and medical equipment supplies.
The NHS also collaborated with researchers at Cambridge University to launch the COVID 19 Capacity Planning and Analysis System (CPAS) that could anticipate the upcoming demand for masks, PPE, ventilators, and medicines at hospitals.
Telemedicine for symptom management
When it became clear that medics were stretched thin and hospitals turned into hotbeds of infection, telemedicine started to gain momentum, assisted by IoMT (Internet of Medical Things) gadgets like smartwatches, pulse oximeters, or common smartphones with specialized apps.
Telemedicine services enabled doctors to remotely monitor patients that did not require inpatient therapy. For instance, Mayo Clinic and Baptist Health, an NPO from Kentucky, adopted the practice of remote monitoring. In collaboration with Current Health Ltd, they will monitor patients with mild and average COVID-19 cases.
Apple Inc. teamed up with the White House Coronavirus Task Force, the Centers for Disease Control and Prevention (CDC), and the US Department of Health and Human Services (HHS) to release the "COVID-19 app", where patients could provide information on their symptoms.
Also, doctors began to open and close sick leave certificates remotely: this practice was introduced in several countries, including Russia.
During the pandemic, the telemedicine approach was actively used for treating patients highly susceptible to COVID, e.g., people with diabetes, HIV, mental disorders, epilepsy, or cancer. To prevent diabetes patients from contacting the coronavirus, they were offered a remote service for instant and continuous monitoring of glucose levels that could be accessed by their therapist. Other services enabled post-surgery monitoring of vital indicators and symptoms among aged cancer patients.
Helping study and treat the coronavirus
Big data and AI also helped scientists understand the specifics of the new virus and its effect on the human body. Researchers at New York University and Columbia University partnered with two Chinese hospitals and developed an AI tool that predicted which COVID patients would experience more severe symptoms. The service also found the impact of liver enzymes, myalgia, and hemoglobin levels on patients' health deterioration.
Together with Amazon Web Services, UC San Diego Health designed and developed the solution for analyzing lung imaging and detecting patients with higher risks of pneumonia.
Vaccine and drug development armed up with big data technologies as well. Google DeepMind used its AlphaFold system to predict protein structure based on large sets of genomic data, helping researchers better understand the virus. In the UK, AI was involved in finding highly effective compounds for COVID-19 treatment and predicting successful drug interactions that could also improve the immune response.
Superfast vaccine launch
During the coronavirus pandemic, among other things, innovative medicines were developed and registered with unprecedented speed. That was how the first two clinically approved mRNA vaccines against COVID-19 were launched, one produced by Moderna and by Pfizer and the other - by BioNTech.
Just a few days after Chinese scientists published the gene sequence of the new virus, Moderna specialists from Massachusetts prepared the plan for vaccine development. 42 days later, they delivered the first batch of the initial vaccine version to the US National Institutes of Health for Phase I trials. The vaccine was tested on people for the first time in early March, even though medication development generally takes years or even decades.
By the way, mRNA technology has a relatively long history: similar methods have been used in cancer studies since 2011, while the first research on mRNA dates back to the 1990s.
As for oncoimmunology, RNA molecules demonstrated promising first results for cancer gene therapy. Moderna scientists working on an mRNA vaccine personalized for each patient based on their specific tumor got positive interim results for patients with inoperable head and neck squamous cell carcinoma.
Fragments and leaks
Unfortunately, despite vast amounts of data and numerous analytical tools that became available over recent years, terabytes of data and their applicability remain an issue.
Scientists complain that technologies implemented by healthcare systems of several countries (or even one country) are siloed and fragmented. The absence of cross-system compatibility leads to the fact that collection, logging, distribution, and exchange of medical data between hospitals and state authorities, let alone different healthcare ministries, can be done only manually. Besides, data arrays that differ in size and characteristics are extremely difficult to consolidate in a single spreadsheet.
Researchers point out that apart from COVID-19, human history will witness many other emergencies requiring a prompt government response. That means data exchange initiatives and data conversion systems already need to be promoted and developed.
Other concerns over the widespread application of big data and AI technologies are related to personal data security and confidentiality of personal health records. Human rights activists have reasonable doubts that private information would be used solely for epidemiological reasons, as data leaks are happening more and more often.