How AI Supports Financial Institutions for Deciding Creditworthiness Artificial Intelligence (AI) emerges as the most accurate, instant, and practical method to check the payback abilities of borrowers.
Opinions expressed by Entrepreneur contributors are their own.
You're reading Entrepreneur India, an international franchise of Entrepreneur Media.
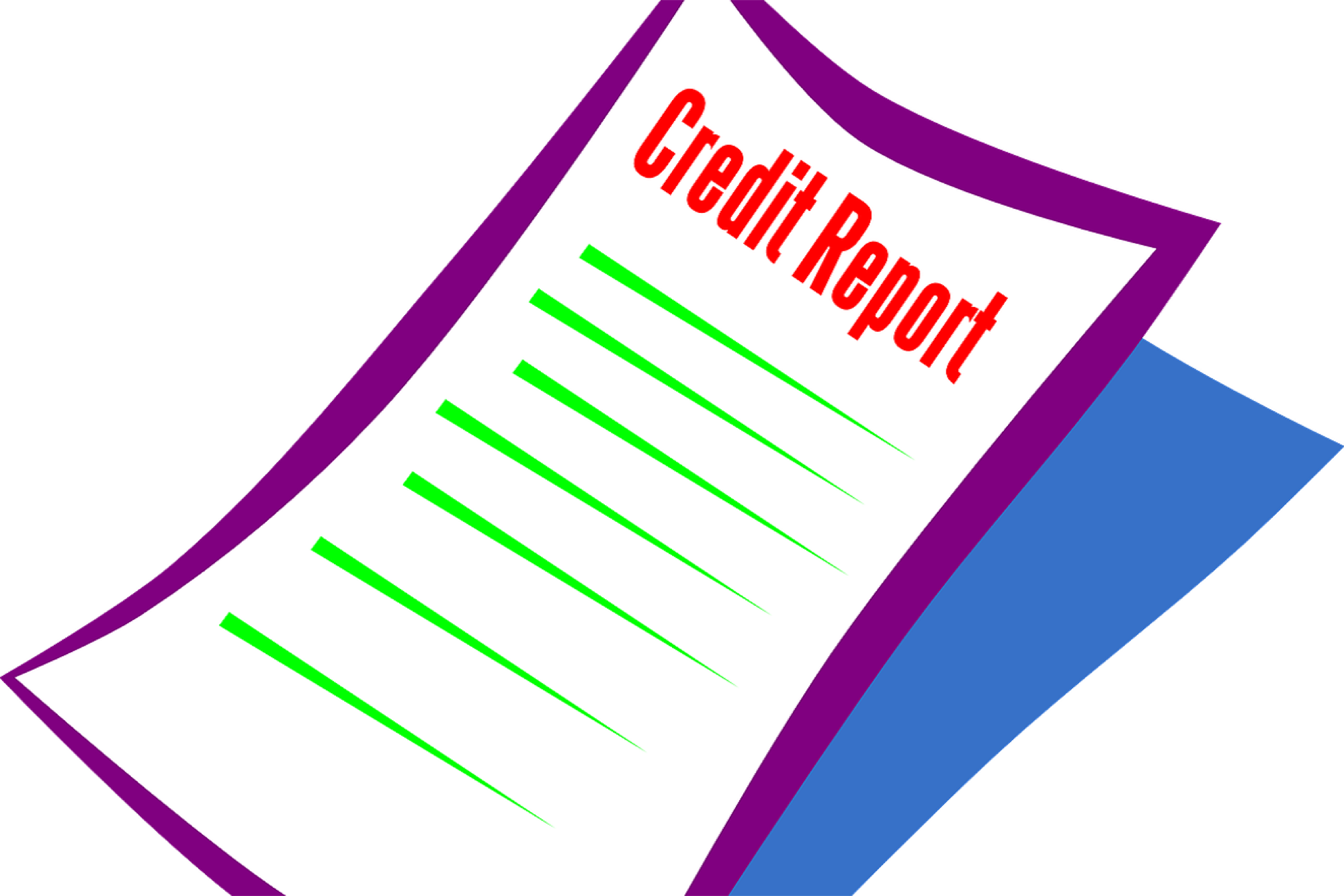
Various macro and microeconomic factors govern the financial institutions and many of the factors are responsible for bringing these institutions closer to risks. Lending is one of the key operations of banks and NBFCs that generate ample revenues for them, but loans cannot be sanctioned to anyone.
Usually, financial institutions provide loan depending upon the creditworthiness of prospective borrowers through CIBIL Score, but in many cases, a large number of potential borrowers deprive of loans due to unimpressive credit history, but it doesn't mean that they come in the list of defaulters. Ignoring these customers is not in the favor of lenders, too, because they lose business.
On the other hand, industries like real estate, education, health, and automobile also bear the brunt of it because the money was expected to be spent in these sectors. On the whole, strict CIBIL Score is not the ultimate way to decide the creditworthiness of loan seekers, and to overcome its demerits, Artificial Intelligence (AI) emerges as the most accurate, instant, and practical method to check the payback abilities of borrowers. The digital credit evaluation system is no doubt the turning-point of the industry.
How it Works
Earlier, credit providing scenes majorly involved the credit scores to make lending decisions for firms and clients. Different loan providers rely on different credit models, but at their core, all these models are laid on the foundation of the borrower's transaction history and payment details from financial institutions. Other than that, these models calculate the credit score from the limited amounts of structured data provided to them by tools like regression, decision trees, and statistical analysis.
However, banks and other financial institutions are now adopting a more holistic approach in credit providing process by also including the data from additional, unstructured and semi-structured sources, like that of social media activity, mobile phone use and text message activity, and improve the rating accuracy of loans. There are credit scoring tools in today's market that apply machine learning to enable assessment of even the qualitative factors such as consumer behavior and willingness to pay. This ability has paved way for greater, faster, and cheaper segmentation of borrower quality thus, ensuring a quicker and much accurate credit decision, at last.
Greater Access to Credit
The use of machine learning algorithms is not just limited to creating a precise, segmented assessment of creditworthiness but, has also enabled a greater access to credit. In most of the traditional credit scoring models, the potential borrower was required to have a sufficient amount of historical credit information available to be considered "scorable.' And, in its absence, the potentially creditworthy borrower often got denied for credit as the credit score can't be generated. Thankfully, with the help of alternative data sources and the AI systems, lenders can now arrive at credit decisions by assessing the ability and willingness to repay, that had earlier been an impossible task to achieve.
A Paradigm Shift
Past few years have witnessed a host of FinTech start-up companies emerging and serving the needs of customers who weren't able to get the assistance of banks under the traditional credit system. The use of AI has brought both advantages and disadvantages in the credit scoring models, like the initial phase of any other technology would do. But, thankfully the advantageous pattern of AI is much powerful than the disadvantageous phase.
With AI by their side, financial institutions are able to analyze massive amounts of data very quickly thereby resulting in credit scoring policies with the potential of handling a broader range of credit inputs, lowered cost of credit risk assessment for individuals and increasing the number of individuals for whom credit risk can be measured. An example of the application of big data to credit scoring could include the assessment of non-credit bill payments, such as the timely payment of cell phone and other utility bills, in combination with other data.
Risk minimization for lenders
Machine Learning can do what humans usually get failed to do. And, a perfect example of this is accurately identifying the rogue investors working across multiple accounts; machine learning does this by deploying predictive analysis to huge amounts of data in the real time. Bringing the AI in their efforts to digitize credit risk processes can help the banks tap on the nearer-term gains while building a key capability for the overall transformation. Machine learning can be applied in early-warning systems (EWS), for example; bringing deeper insights at the desk from the large, complex data sets, without fixing the limits of standardized statistical analysis.
With a machine learning–enhanced EWS, financial institutions get enhanced in automated reporting, portfolio monitoring, and recommendations for potential actions, including an optimal approach for each case in workout and recovery. While the debtor finances and recovery approaches gets easily evaluated, the qualitative factors, on the other hand, gets assessed automatically on the basis of large volumes of nontraditional (but legally obtained) data incorporated in the systems.
Expert judgment is embedded using advanced-analytics algorithms. In the SME segment, this institution achieved almost 70 to 90 percent improvement in accurately predicting late payments six or more months prior to delinquency.