Credit Access to SMEs: Utilizing AI to Enhance Credit Lending for Banks Several fintechs have been successful in providing an automated application and decision-making process for smaller and more commoditized loans
Opinions expressed by Entrepreneur contributors are their own.
You're reading Entrepreneur India, an international franchise of Entrepreneur Media.
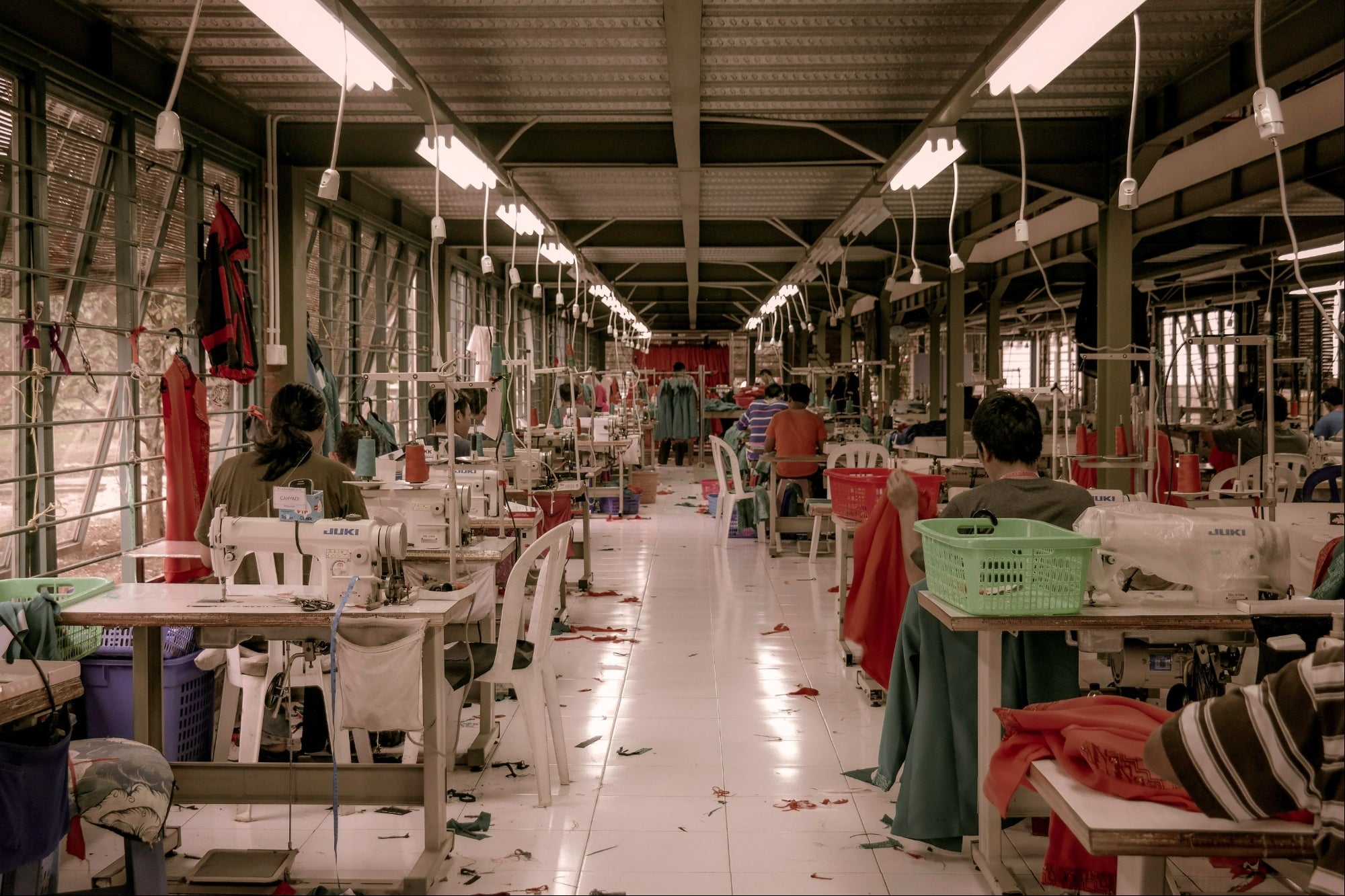
Progress in artificial intelligence (AI) and automation has improved many parts of financial services – in particular in retail banking where for example, low-friction, highly automated customer offerings have been identified as one of the most important emerging trends in retail banking. These techniques have struggled however, to make inroads in many areas of commercial lending. This is because of the relative unavailability of sufficient data, and because most banks still use a decision-making process that is largely manual.
Several fintechs have been successful in providing an automated application and decision-making process for smaller and more commoditised loans such as simple working capital facilities. As loans become larger and more complex however, it becomes harder to make informed credit decisions at speed and at scale, in a cost-effective manner. As such, most commercial banks today still use processes that have remained largely unchanged for the last few decades. In order to improve these, the focus has primarily been on digitising existing processes to move away from paper, rather than radically altering the process to allow for more efficiency.
One of the challenges facing any attempt to improve this is the availability and quality of data. While public data is generally available for large borrowers who have listed equity or debt, for smaller borrowers, information is typically hard to come by. Even if that was not the case, any attempt to build an automated decision process for commercial lending along the lines that have been so successful in the credit card industry, for instance, would rapidly hit the limits of available data, given how narrow and specific are the decision-making criteria for commercial lending.
To build decision models for high-volume retail loans, a typical approach is to score each loan using a standardised credit score and then build a Markov state transition model. This divides loans into states of default (from 'current', through '30', '60' and '90 days delinquent' to 'defaulted', for example) and then estimates the probability of a loan to a borrower in a given credit score band moving from one category to another. For commercial lending, however, even a large lender is likely to have a few hundred thousand loans at most, and once you divide these by sector, size and vintage of loan, revenue size of company and region, you very quickly get to a number of loans in any given bucket that is way too small to estimate the probability matrix. How, then, can it estimate a probability of default for these loans? In addition, if you make an estimation error when fitting a model for small loans, the resulting losses are by definition, also small. As the loan sizes increase, the potential losses increase too, and rapidly approach a point where an unexpected event affecting several borrowers could have a meaningful impact on the capital of a small bank.
There are a number of ways in which AI and automation can help with these challenges. First, we can use statistical techniques to create forward-looking analysis. For example, if we are considering a loan in a given sector, we can look at macroeconomic variables and use those in an attempt to predict the possible future revenues, costs and market size in that sector. This in turn can be used to make intelligent sector-specific scenarios that can be used to predict borrower performance and understand the borrower's future financial needs and risks. Driver analysis can then use statistical methods to identify relevant drivers for a target time series among all these potential macroeconomic variables. As additional data sources become available, we can use similar analysis to determine whether any relevant drivers are present in these new sources and whether or not the new sources would be additive.
A related technique is nowcasting, which uses the techniques of prediction to fill in the gaps between the last reliable historical data points and the present time, allowing a bank to effectively eliminate the problem of data source lag and understand the current state of its portfolio and borrowers. Utilising high-frequency datasets, nowcasting predicts the current value of key sector-level indicators such as sector revenue. These predicted indicators can serve as an early indicator, bringing attention to the potential risks developing in a portfolio. The statistical methods used — which include back testing and regression — provide confidence intervals that can help evaluate the accuracy of the predictions.
The purpose of AI and automation in commercial lending is not to replace humans in the decision-making process, but rather to augment the capabilities of the human to enable them to make better, smarter decisions and to operate with greater speed and efficiency. If systems can automate sector, peer, and financial analysis, produce intelligent insights, and highlight borrowers who may have difficulties in the future, as well as those where there may be opportunity for growth, we will be able to make better decisions that lead to better credit outcomes, and ultimately create a better borrowing experience for businesses.