4 Building Blocks of the In-House Data Science Team You Need Know what skills are missing and who can provide them.
Opinions expressed by Entrepreneur contributors are their own.
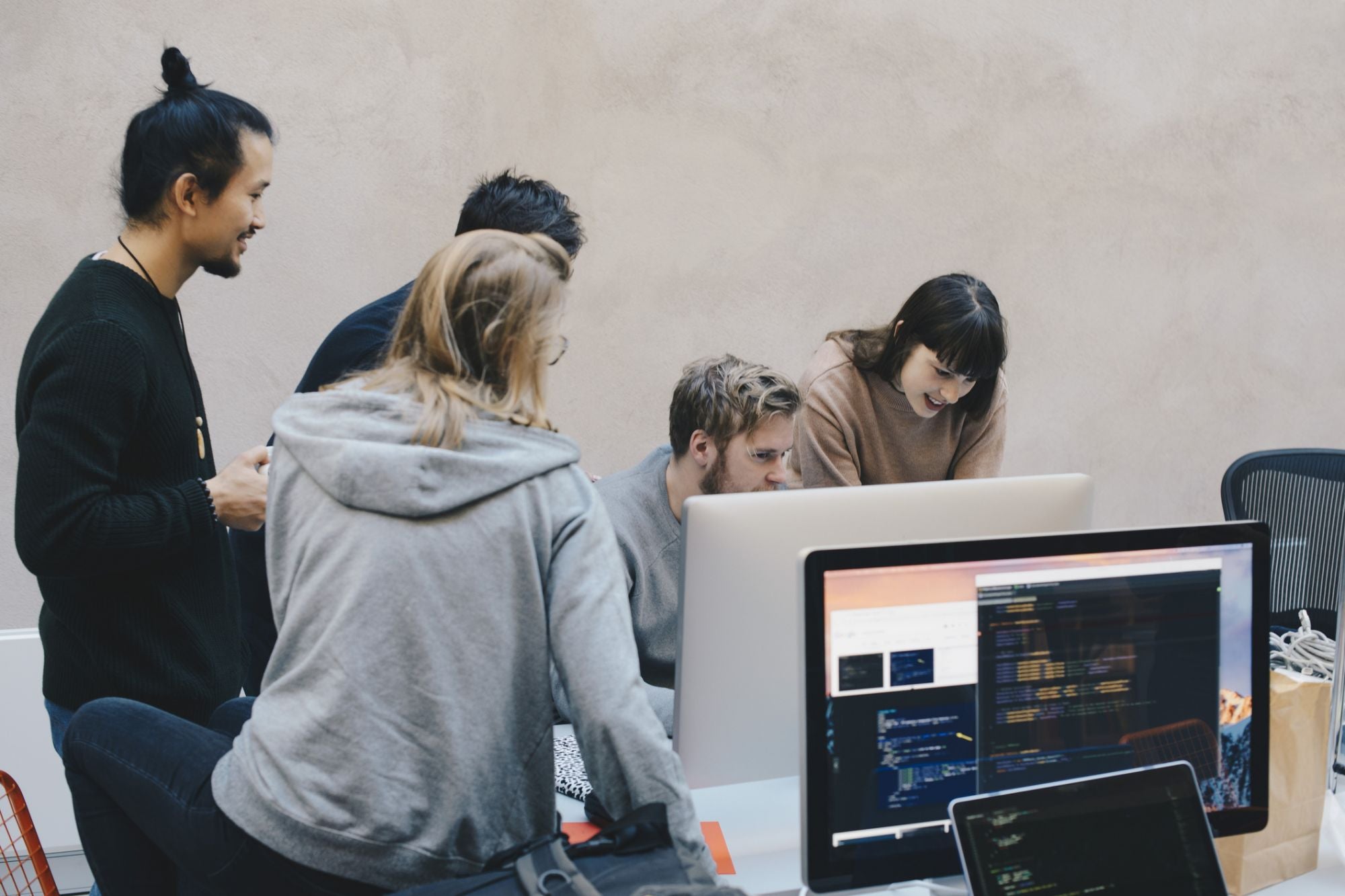
You've been grinding for months to recruit data scientists to your company to build some excellent machine learning capability into your hardware or app. You have one, or two, or 10 on the team, but you feel like you need more. If you're not already, you should be thinking about how to keep these hard-earned talents focused, happy and motivated. Here are a few pieces of wisdom I have culled from years of managing teams of data scientists.
Know the roles.
"Data scientist" has become a pretty vague term that people frequently misuse to label all kinds of talents. The first thing to do is familiarize yourself with the specific skills that exist and the skills you want on staff. On bigger teams where you're doing your own infrastructure and basic research, what you want is some combination of data scientists, data engineers and machine learning engineers. The disciplines overlap but significantly differ in their purer form.
The data scientist is an applied mathematician at heart. Their greatest strength is designing the right questions to pose your information. What makes them happiest is solving problems, not necessarily spending hours writing software, even though programming is one way to do that. The data engineer is the specialist who gets the information ready for analysis, dealing with plumbing, error logging and scaling up a fast infrastructure. The machine learning engineer is a hybrid specialist who combines the modeler's artistry with the software engineer's ability to put that art into production. If your urgent need is to put models into production, hire machine learning engineers. But it's good to know the differences if you want to build a bench with various talents.
Related: Think Your Company Needs a Data Scientist? You're Probably Wrong.
Hire for raw horsepower.
If you want to avoid the hire-fire-rehire cycle, select people with malleable talent over people with tons of experience in one discipline. No one is going to be able to do everything, but a great team, especially at smaller startups, should be able to swap hats as and when needed. Where one employee has less knowledge and experience, another can support and help that employee grow.
Find a balance between research and applied science.
Just as machine learning models are built to learn continuously, so are data scientists and machine learning engineers. Data science and modeling are at the forefront of mathematics, and the field frequently evolves as discoveries emerge. A significant portion of a data team's knowledge goes obsolete on an annual basis. That's why we offer our team yearly stipends to attend conferences and take online courses.
Encourage your folks to publish and participate in open source projects. That said, a data science team can only help your startup succeed by applying their knowledge to solve business goals. A lot of science projects turn out to be duds. Our modeling team runs a book club-style group to help weed out good ideas from the lame ones. One team member highlights an article or new technique and presents it to the whole data science team. Another person tests it out on our "sandbox" of data sources and presents the findings. This way, the rest of the data science team isn't getting sidetracked.
Related: Deep Learning and 'Hyper-Personalization' are the Future of Marketing Automation
Provide a clear career path.
The most important thing you can do as a founder/CEO is keep great people happily planted in your organization. That means helping new hires find a career path. There are lots of ways people may want to grow. For example, some of our modelers have become great partners to salespeople on the road meeting with customers. Some could easily shift over to the business team and do front-line analysis of new client data before it hits the modeling team. Some may want to go deeper into technical expertise, and those folks can train what you would hope is a larger team in a few years. Whatever keeps them happy and productive, growing like a weed.