Me, Myself and AI: Is That My Privacy in the Rearview Mirror? Mountains of data are what make machine learning possible, the whole project is dead in the water without it. But whose life is it, anyway?
Opinions expressed by Entrepreneur contributors are their own.
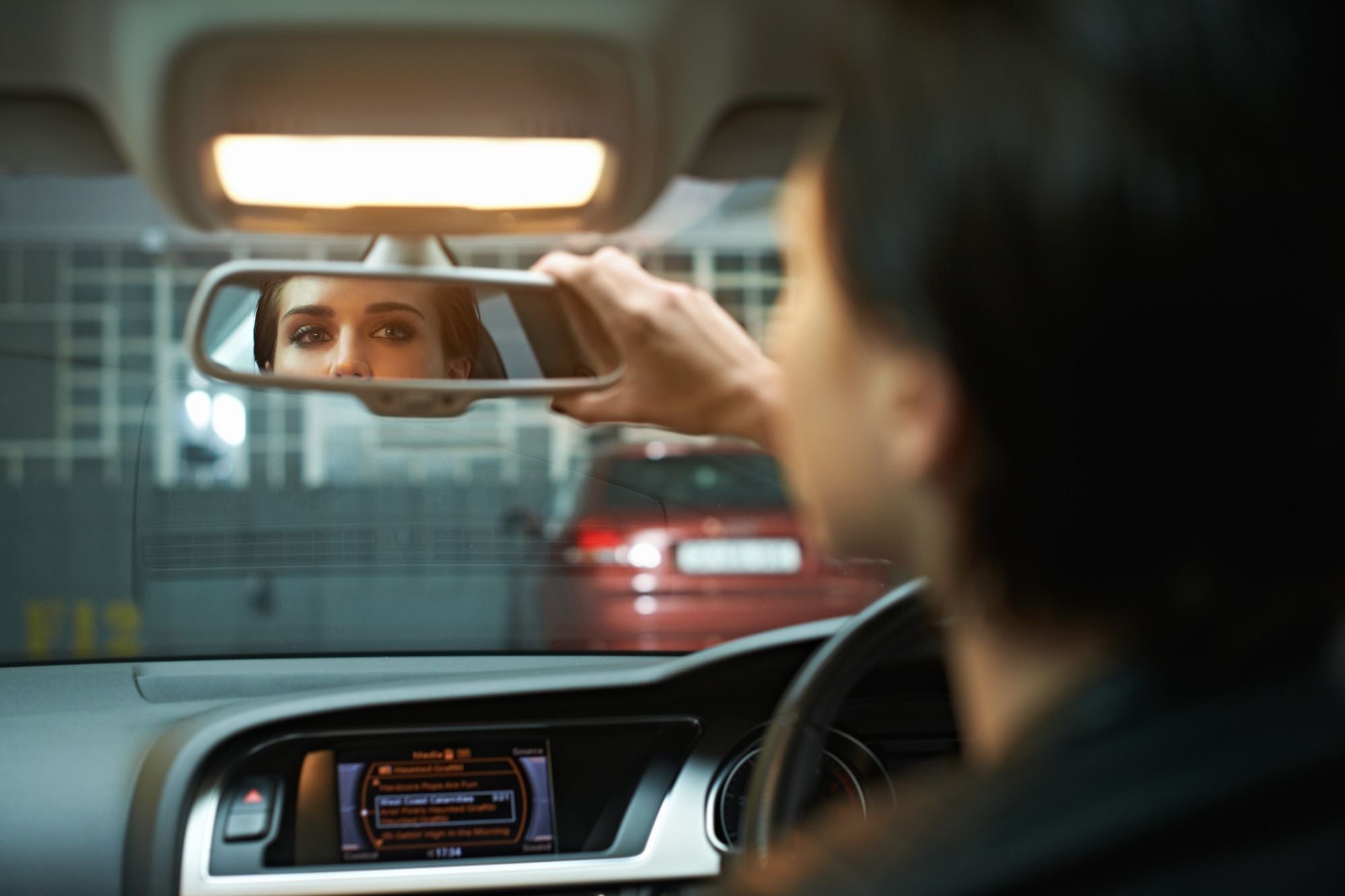
I had the pleasure of meeting Sophia in London a few weeks ago. Sophia is a popular, outgoing personality that looks a little bit like Audrey Hepburn. As it happens, Sophia is also a machine. What makes her interesting is that she can carry a conversation. She listens to what you say, shows facial expressions as she speaks, answers your questions, and even asks follow-up questions of her own.
Sophia is just one of many examples how far machine intelligence has come over the past few years. Even if the use of robots as the primary user interface is still rare, real-life applications of artificial intelligence (AI) in image processing, speech recognition and natural language processing are now commonplace.
The groundwork for Sophia and other AI demonstrations was laid back in the 1940s and 1950s, during early work on cybernetics, computation and artificial neural networks, and through the development of machine learning algorithms.
Catching up to mankind.
While the field has progressed in fits and starts over the last few decades, things are now coming together. For instance, it was thought that beating a human master in a game like Go would be beyond the capacity of AI, given that the winning strategy cannot be found with brute-force computing. As it turned out, AlphaGo (created by DeepMind, acquired by Google) beat the Go world champion Lee Sedol 4-1 in a five-game series two years ago, while seemingly exhibiting very human characteristics like intuition.
Rapid progress is being made in AI for a few reasons. The availability of large-scale computing fabric such as cloud computing as well as fast stand-alone supercomputers, alongside significant theoretical progress on machine learning algorithms, means we can now do things that were impossible before. However, training a useful and realistic system can take hours, days, or even weeks, depending on what you're running on. Still, AI applications which in the past were simply unfeasible can now be tackled.
Grist for the AI mill.
But training AI algorithms isn't simply about computing power. Possessing relevant data is the key to making further progress. Much of AI involves machine learning where automated methods are used to find patterns in large data sets, to classify objects, and to make predictions of what will happen next. In some tasks, machines -- after being shown lots and lots of examples, that is, data -- already perform much better than anyone of us could ever hope to.
Luckily, we live in an era where data in sufficient varieties and volumes is now readily available. The ubiquity of smartphones, connected devices, home or garden robots, and the exponentially growing number of sensors around us means that massive amounts of information are being collected about human beings, from our location, health, residence and our demographic profile, to financial transactions and our interactions with others.
However, much (if not all) of this data is inherently personal. That personal aspect is what necessarily raises issues of privacy and trust.
My data, my life.
Is my privacy being respected, or is personal data being collected without my consent? Who is doing the collection and how? Is the personal data being stored securely? Does the data stay as my own personal intellectual property? Is the raw data, or the knowledge derived from the data, being made available to the authorities and to the government, either my own or another one?
Related: Until We Ban Data Brokers, Online Privacy Is a Pipe Dream
Events like Cambridge Analytica allegedly amassing Facebook data in underhand ways have brought these issues into the open. Again, recent stories like Amazon's Alexa recording a private conversation and sending it to a colleague surreptitiously are alarming. Once we start employing a multitude of devices in our homes, all listening to commands and even giving instructions themselves, there's potential for even deeper confusion and privacy concerns as machines start having conversations among themselves and entering into commercial transactions with one another.
In addition, what would be the incentives for ordinary people to share their personal data? In some cases, I might want to share information without any compensation if doing so benefits my community or the common good. I might also be willing to share data if in return I get access to new services, or if some existing service is improved with more data.
Sharing is caring?
This is conceptually what is already happening for users of say, Google Maps. Phones and other connected devices track our geolocation, speed and heading. When such information is aggregated and sent back to route-finding algorithms, a better picture of real-time traffic flows emerges. Users share their data for free but receive an even better functioning service in return. Google, of course, makes massive profits from serving ads to those same users and knowing far more about them and their habits than they could otherwise dream of.
There are many other services offered by big companies like Amazon or Facebook which don't give their users much practical choice of whether to share their data or not. In China, the web is far more centralized than in the West, and large companies like TenCent or Alibaba routinely collect data from their users (and share it with their government, too).
Related: Google Reportedly Working on Censored Search for China
In the more general case, however, tangible economic incentives are needed to encourage people to share. If people could be reassured that their privacy would be respected and there was a monetary reward for sharing their personal data, wouldn't they be even more likely to entertain the possibility of doing so?
Let's go back to Sophia for a moment. She is still primitive in many ways. But she represents an attempt to go beyond weak AI, i.e. machine intelligence that is limited to narrowly predefined tasks or problems. Unsurprisingly, strong AI is the new holy grail, one that exhibits general intelligence. The goal is to create conscious, self-aware machines capable of matching or surpassing human problem-solving capabilities.
Fast track with no guardrails.
Of course, we haven't yet mastered how to build such machines, but if nature is our inspiration, neuroscience shows that intelligence is very much a product of our life experience. From birth, our brain is molded and connections pruned on the basis of interaction with and feedback from other people, and our environment.
The prospect of increasingly powerful machine intelligence raises the importance of the quality of the personal data that is being fed to AI models. A machine can only learn from the information given to it. If the input data is biased, then models based on such data will lead to biased predictions and decisions. A good example how badly this can go is Microsoft's chatbot (Tay) which quickly learned -- based on a right-wing tweet barrage directed its way -- to become a racist, alt-right entity. There are no good mechanisms in place to ensure the objectivity of input datasets, which presents a worrying challenge in and of itself.
Related: Microsoft Apologizes for Chatbot's Racist, Sexist Tweets
At some level, what we are seeing in AI is a reflection of competing Internet worldviews, according to Frank Pasquale. On one side, you have the centralized or Hamiltonian ideal with data collected and utilized by large enterprises to build ever better AI models. On the other side, you have a Jeffersonian view where decentralization is seen as a way to promote innovation and where people retain control over their own personal data and share it on their terms with the AI community. Which one is better? Time will tell.