The 5 Biggest Mistakes When Hiring Data Scientists Because of their high demand, the process of hiring data scientists needs to revisited
By Sham Mustafa
Opinions expressed by Entrepreneur contributors are their own.
You're reading Entrepreneur India, an international franchise of Entrepreneur Media.
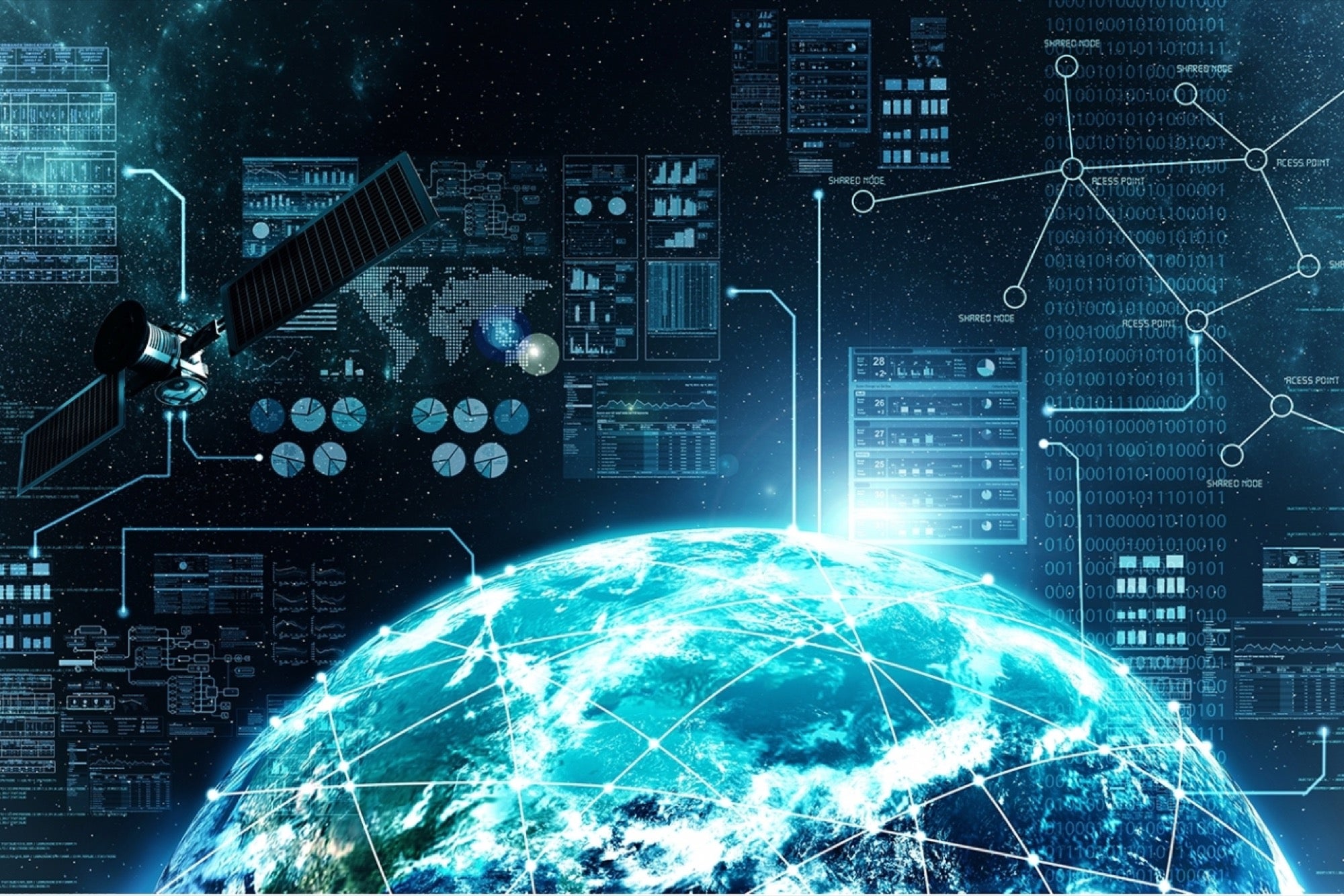
Data scientists are in high demand as employers of all sizes and in every industry from retail to gaming are building up their own data science teams. Over the next few years, demand for data scientists is projected to exceed supply by more than five times. Unfortunately, employers have to spend a lot of time and money recruiting top talent, and many employers often make mistakes that keep them from successfully building out their data-analytics team.
Below we'll highlight five of the most common pitfalls to avoid as well as several solutions that will provide insights into how one can improve the hiring process.
Imprecise Job Title
A good job post begins with the right job title. Employers often use "Data Scientist" as a catch-all title, but what are you really looking for? Is it someone to build analytics dashboards and track key metrics? Someone to create prediction algorithms? Someone to develop your data ingestion workflow?
There is a world of difference between a Machine Learning Engineer, a Big Data Developer, a Business Intelligence Analyst, a Quantitative Researcher, a Predictions Algorithm Developer, a Customer Success Manager, a Data Engineer, and so on.
A general job title may result in more responses but being more specific in your job title will in the end save you time when sorting out those that do not fit your needs and help you concentrate on finding the exact type of talent that you need.
Tip: Craft your job title to most accurately reflect the role.
Failing to Emphasize Interesting Problems
The best data scientists are attracted to interesting problems as well as to the latest algorithms and technology tools. Rather than emphasize benefits or pay, emphasize the intellectually interesting components of the business problem you need solved.
This might mean talking up the natural language processing, computer vision, fraud detection or deep learning involved, as well as the use of big data tools like Spark. Speak to the opportunities of making ground breaking advancements in technology that will potentially position them as the leading experts in your field.
Be sure to emphasize the team that the data scientist will be working with and the opportunity he/she will have to learn from others who have expertise in complementary areas. Present the opportunity not only as a way for them to move your company forward but also as a way for them to learn and work together with others to create advancements that would not have been possible with teams at other places.
Tip: Emphasize interesting problems and technology rather than benefits and pay.
Defining Experience Too Narrowly
The term "Data Scientist" was coined seven years ago, and has only become a more mainstream job title in the past couple of years. When employers look for an experienced Data Scientist, they often want to see someone who has had the job title "Data Scientist' for a few years. This is a mistake. Many researchers in quantitative finance, biostatistics, high energy physics etc. are doing data science work every day but don't go by the title "Data Scientist'. This population represents a vast pool of underutilized supply for employers seeking experienced Data Scientists.
Take time to have in depth conversations with potential candidates that go beyond talking about specific needs for the position. Many times you'll uncover subject matter that they have worked on , which could prove to be the seed for an entirely new category of products for your company.
Tip: Candidates can be experienced Data Scientists without having the job title "Data Scientist".
Undifferentiated Sourcing Strategy
Qualified data scientists are in high demand and short supply. A well thought-out sourcing strategy that will attract the right talent pool is essential. It is important to build brand awareness in the data-tech community, for example by speaking at relevant data conferences or by sponsoring hackathons and "datathons." A good sourcing strategy takes time to carry out, so invest in it now ahead of an acute hiring need. You'll not only create an early pipeline but help position your company as an advocate for the data science industry which helps tremendously when candidates have to choose between your offer and a competitor's.
Tip: Go beyond posting the job on your website and jobs boards.
Inconsistent Skill Validation Process
Research shows that interviewing alone is not reliable to validate the skills of candidates. Instead, employers should develop a data science challenge that all applicants are expected to complete. The challenge should represent a real business problem that simulates a-day-in-the-life of the job as much as possible. Candidate submissions should be scored consistently and quantitatively. The result is an apples-to-apples comparison across candidates from diverse background, and a data-driven tool for funneling only the highest performing candidates to interview. These types of challenges also demonstrate your unique company culture and how exciting/rewarding it could potentially be to work at your company.
Tip: Build a skill validation process that simulates a-day-in-the-life of the job rather than focusing on testing academics.
Hiring data science talent is hard. It takes a great deal of time and a unique strategy to find qualified talent, but there are things employers can do to make their internal processes and evaluations more effective to avoid these common five hiring mistakes. When you adopt these strategies, you'll set your company up to build a fruitful pipeline of potential candidates and improve your efficiency in hiring talent – which will essentially be the future growth engine for your company.