Walking With AI: How to Spot, Store and Clean the Data You Need The best time to design your AI initiative is now.
By Sourav Dey Edited by Heather Wilkerson
Opinions expressed by Entrepreneur contributors are their own.
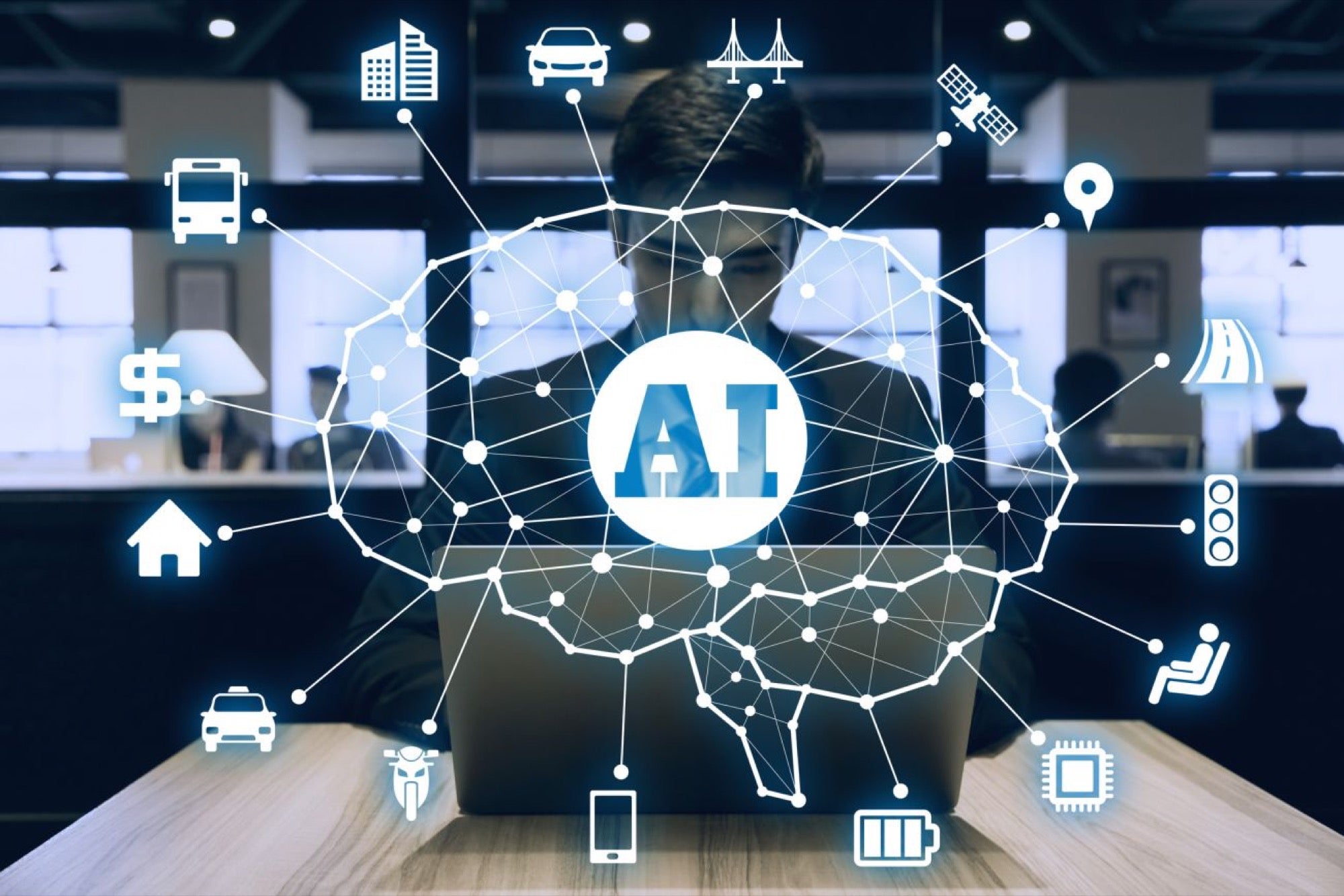
Last August, data science leader Monica Rogati unveiled a new way for entrepreneurs to think about artificial intelligence. Modeled after psychologist Abraham Maslow's five-tier hierarchy of psychological needs, her AI hierarchy of needs has become a conference favorite for illustrating how to incorporate AI into a business.
Despite entrepreneurs' excitement around AI, Rogati's hierarchy makes an uncomfortable point. Few companies are ready to adopt AI. Most are struggling to fulfill fundamental needs, such as reliable data flow and storage. The truth is that data literacy is lacking at most companies hoping to reap the rewards of AI.
You get out what you put in.
To help entrepreneurs understand the importance of high-quality data, our team has come up with what we call the AI uncertainty principle:
The key takeaway? If any of the values on the right fall to zero, so does the value of the AI program. We discussed evaluating business opportunities for AI in a prior Entrepreneur article, so we're now focusing on the second variable: maximizing data quality.
Related: 5 Things to Keep in Mind When Using Data for Artificial Intelligence
High-quality data is key across all types of machine learning -- supervised, unsupervised and reinforcement learning. For most businesses, supervised learning is the low-hanging fruit because it's about learning from past examples. If the prior examples are irrelevant or low-quality, then guess what? Any insights derived from them will be, too. Someone without any basketball experience can't just join an NBA team -- at least not if he wants to succeed.
While most data scientists prefer the hardcore math of machine learning over the legwork of cleaning data, you can't have the former without the latter. Data science and engineering go hand in hand, and the right machine learning team will have people who can handle both.
Related: Think Your Company Needs a Data Scientist? You're Probably Wrong.
Do more with good data; No machine learning initiative will work without high-quality data. To get the good, clean data you need to:
1. Start with instrumentation.
Machine learning initiatives are as diverse as companies themselves. Think critically about what sort of examples you need to train your algorithm on in order for it to make predictions or recommendations.
For example, an online baby registry we partnered with wanted to project the lifetime value of customers within days of signup. Fortunately for us, it had proactively logged transaction data, including items customers added to their registries, where they were added and when they purchased. Furthermore, the client had logged the entire event stream, rather than just the current state of each registry, to maintain a database record.
The client also brought us web and mobile event stream data. Through Heap Analytics, it had logged the type of device and browser used by each registrant into its transactional database. Using UTM codes, the registry company had even gathered attribution data, something collected for all or most marketing activities by just 51 percent of North American respondents to a 2017 AdRoll survey.
Taken together, the logged information enabled the company to record how various marketing campaigns and channels map to customer lifetime value. The only piece it was missing was CRM data on sales touch points and similar metrics. While many of our other clients use CRMs such as Salesforce, human-input data is messy. Although there might be signal in it, we tend to prioritize it below machine-generated data, which is more consistent.
When working with disparate data sets, think about joinability. If you offer a software product, consider requiring a login. Because the registry we worked with used one, we were able to easily associate actions across devices and platforms to a single user. In lieu of a login, which can create user friction, consider logging user IP addresses or using tracking cookies. One way or another, individual actions must be tied together into a single coherent view of the user.
2. Label and store the data.
Store your data in a data warehouse, such as Google BigQuery or Amazon Redshift, though there are other strong storage options. These systems use structured formats that force discipline, which make it easier for downstream users to access and analyze the data.
Build labeling into your storage workflows, and try to automate labeling as much as possible. On one of our predictive maintenance projects, for instance, requiring technicians to use an app for logging failure causes would have produced a clean, labeled data set. Humans are inconsistent both over time and across individuals, and unless you create truly excellent systems for data input, it will be tough to normalize the data for these disparities down the road.
To make normalization easier, cleanly label data lineages and track them alongside the data itself. Product changes can discolor your data in ways that won't be readily apparent to analysts and engineers. If you roll out a new user interface, for example, clearly identify data from before and after the switch.
3. "Clean" the collected data.
Cleaning data is far from exciting, but it's critical if you want to get results out of an AI initiative. When it comes to AI projects, 51 percent of those surveyed for CrowdFlower's 2017 Data Scientist Report called quality issues their biggest bottleneck. Cleaning can involve interpolating missing records, removing outliers that skew results, deleting redundancies and logging regime changes. If you're starting from scratch, cleaning data might involve all these things and more, such as back-filling missing data.
Related: What to Do When Your Mother Tells You Your Data Resembles 'a Rat's Nest'
Remember the AI uncertainty principle. When data is missing, incomplete or dirty, you won't get much value from your AI. That being said, don't pitch an effort to clean all your data in one fell swoop.
With our registry client, we began by working solely with the transactional database and migrating that into Redshift to create a number of downstream models. Only after that did we incorporate the client's Heap data into Redshift, and we're currently doing the same with its email marketing data.
If you're not sure where to start, pick an end-to-end solution that delivers business value with the added byproduct of data cleansing.
As important as collecting and cleaning data is, know this: It'll never be enough. Just as they have since the start of your business, your products, contexts and goals will continue to change. Your data collection and cleaning efforts should as well. That's why the best time to design your AI initiative was when you began your company; the second best time is now.