The 5 Most Interesting Artificial Intelligence Trends for Entrepreneurs to Follow in 2018 The current pace of innovation makes it almost impossible to stay on top of the AI trends, but understanding these advancements is a must for business owners who want to stay ahead.
By Artur Kiulian Edited by Dan Bova
Opinions expressed by Entrepreneur contributors are their own.
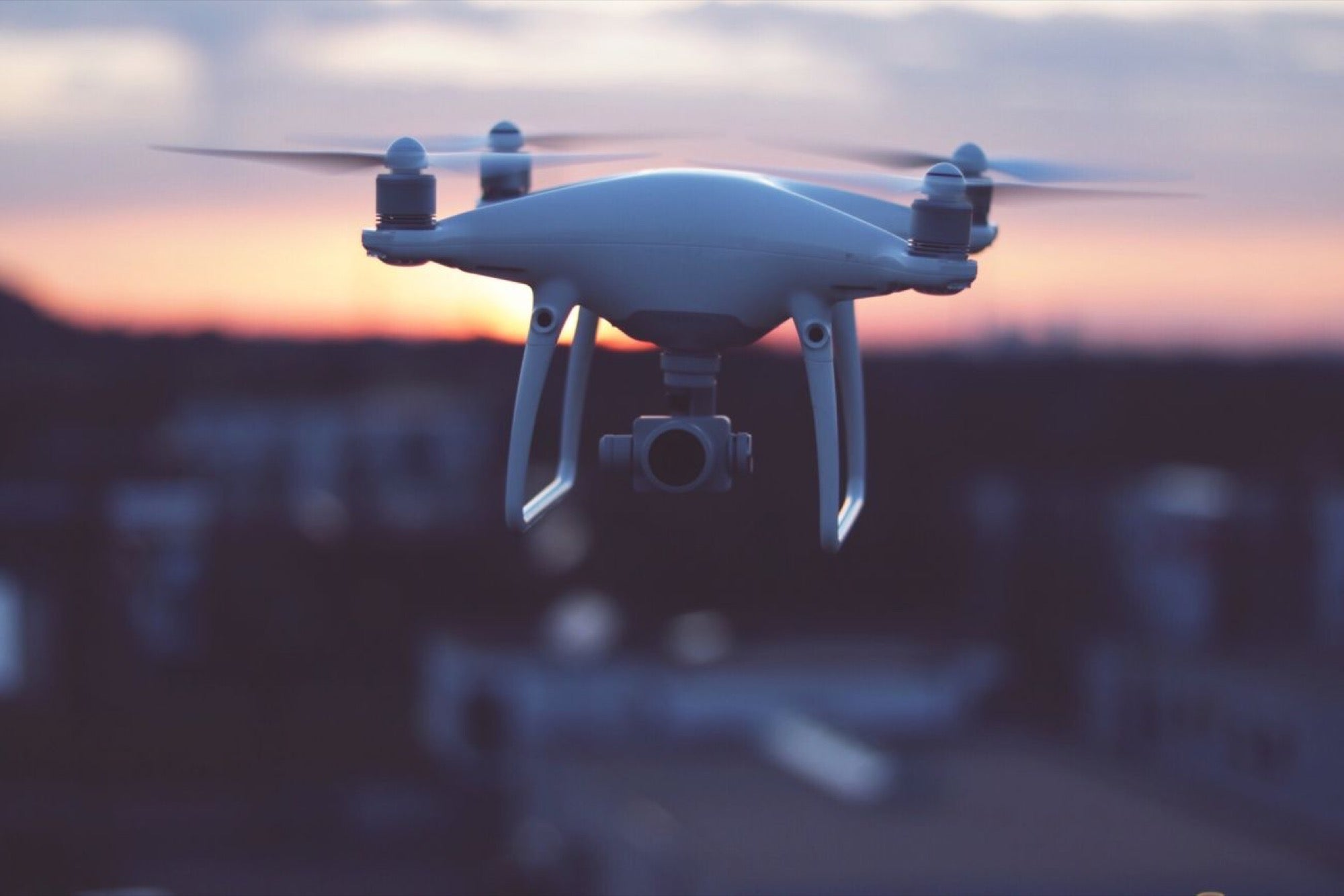
New advances in artificial intelligence (AI) and machine learning (ML) research made by tech giants and academia have quickly made their way into businesses and business models, while even more companies are introducing established AI solutions like chatbots and virtual assistants. Following all that is happening in the dynamic world of AI is time-consuming for entrepreneurs who are busy running their own companies, so I've compiled a list of the most interesting AI trends entrepreneurs should keep an eye on in the coming year.
AI content creation
The trend toward humanization of big data and data analytics will continue in 2018 with new advancements in natural language generation (NLG) and natural language processing (NLP). Using rule-based systems like Wordsmith by Automated Insights, media outlets and companies can already turn structured data into intelligent narratives based on natural language.
Related: How Your Business Can Stay Ahead of the Game With Artificial Intelligence
Making relationships in data understandable to people beyond data science teams will further democratize AI and big data, leading to the era of automatic generation of insights. The same technologies are already enabling automated content generation in news coverage, social media, marketing, fantasy sports, financial reports and more. In the coming year, automated content generation is likely to gain more traction in news reporting and marketing, helping companies instantly respond to emerging trends, news and events by creating the relevant content for their audience and clients.
The rise of capsules AI
Capsule networks (CapsNet) is a new form of deep neural networks proposed by Google's lead scientist Geoffrey Hinton in a recent paper. In a nutshell, a capsules approach aims to overcome the shortcomings of CNNs (convolutional neural networks) that have been the de facto standard in image recognition for many years. CNNs are good when images fed to them are similar to those used during training. However, if they are asked to recognize images that have rotation, tilt or some misplaced elements, CNNs have poor performance. CNNs' inability to account for spatial relationships makes it also possible to fool them by changing a position of graphical elements or the angle of the picture.
Related: 5 Ways to Make AI Work for Your Organization
Conversely, capsule networks account for spatial relationships between graphical elements and understand natural geometric patterns that humans grasp intuitively. They can recognize objects no matter from what angle or point of view they are shot. Commentators predict that capsules will be the next major disruption in image recognition and computer vision. In particular, new capsule networks will dramatically outperform CNNs and other image recognition models and will be able to counter white box adversarial attacks designed to trick neural networks.
Decentralized AI
Until very recently, training of machine learning models was made in a centralized fashion on remote cloud clusters. AI companies had to manually collect large training data sets and feed them to ML algorithms run in data centers equipped with dedicated hardware (e.g. GPUs) for machine learning. The main downside of this centralized model is the difficulty of making rolling updates of AI software and implementing continual training using the constant stream of incoming data generated by users and applications.
Related: Top 3 Ways Machine Learning Will Create Jobs
Decentralized AI can also gather momentum with the development of edge computing that moves intensive computations from remote cloud applications to the network edges where digital devices sensing and collecting information are installed. Moving data processing and analysis to the "field" solves the problem of high latency and low throughput associated with sending data over the network. Many edge devices need advanced learning, prediction and analytics capabilities to function efficiently. This is where AI and ML have an opportunity to shine. Using AI on the edge is especially critical for drones and driverless cars, which need to run real-time deep learning without the network connection to avoid the disastrous or even fatal consequences of network failure.
To close the existing gap in AI for the edge, companies like Movidius (acquired by Intel in 2016) are creating AI co-processors and edge neural networks that can be used for obstacle navigation for drones and smart thermal vision cameras. In the coming year, we are likely to see more innovation in low-power computer vision and image signaling hardware and software specifically designed to enable AI on edge devices like security cameras and drones.
Related: 10 Ways AI and Chatbots Reduce Business Risks
AI leveraging offline data
Data generated online is currently one of the main sources of insights for data analytics and AI-based solutions. However, major retailers like Amazon have already ventured into an unchartered territory of offline data collected by small digital devices like sensors and actuators in stores and malls. In Amazon Go grocery stores, for instance, these devices already track customers' movements to see how long the customers interact with products. Data collected by Amazon sensors is stored in the Android app and Amazon account, which are required to shop in Amazon Go stores. In this way, Amazon accumulates mountains of data about consumers.
Using this data, AI algorithms can draw insights about consumer preferences and behavior to create automatic price-setting mechanisms and introduce more efficient marketing, product placement and merchandising tactics. Sources of offline data, however, are not limited to grocery stores. Using drones and the internet of things, AI companies will gradually transform the entire physical space we live in into a giant source of data for ML algorithms and models.
Related: Could AI Developments Get Out of Hand? Have They Already?
The rise of on-device AI: Core ML
Running AI software or training ML algorithms on mobile devices has been recently regarded difficult due to battery power constraints and limitations of mobile computing power. In 2017, however, we witnessed the move towards on-device and mobile AI heralded by CoreML, Apple's ML library designed for iOS 11.
CoreML ships with a variety of trained ML models (e.g. for image recognition, text detection, image registration and object tracking), which can be easily integrated into iOS applications. All models are optimized for efficient on-device performance using low-level Apple technologies like Accelerate and Metal. As a result, iOS developers now have a powerful ML functionality at their fingertips, which promises to make AI/ML apps mainstream on mobile devices in 2018.
The current pace of innovation makes it almost impossible to stay on top of the AI trends, but understanding the terminology and the applicability of the machine learning advancements becomes a must for business owners in 2018. Using this knowledge, entrepreneurs can navigate the landscape and truly benefit from the improvements, even if they seem to be incremental.